Comment l'apprentissage automatique s'applique-t-il aux agents virtuels ?
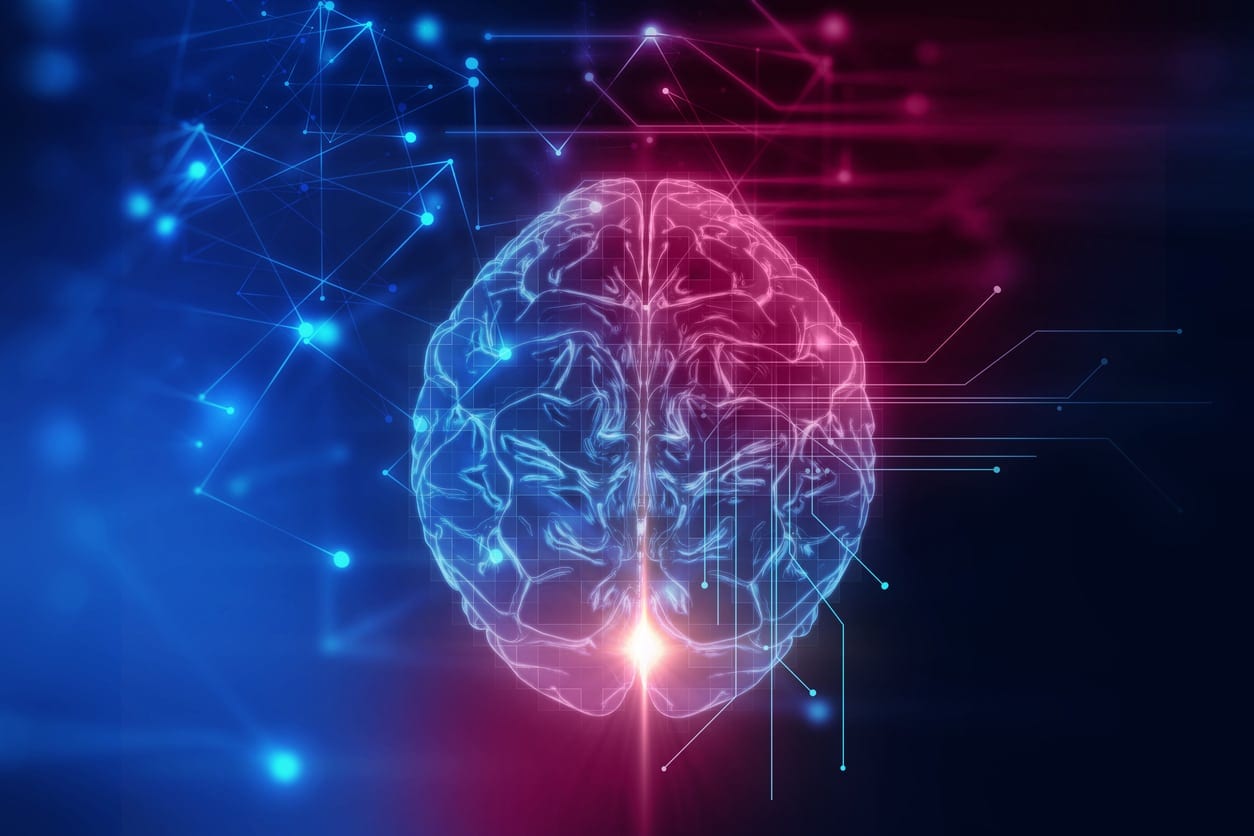
L'apprentissage automatique est un vaste domaine dont l'importance s'est considérablement accrue au cours des dernières années. Il joue désormais un rôle clé dans les performances des agents virtuels. Cependant, la relation entre l'intelligence artificielle, l'apprentissage automatique et l'apprentissage profond reste confuse. Revenir à une définition claire est un bon début.
L'intelligence artificielle en quelques mots
L'intelligence artificielle est devenue une discipline universitaire officielle au milieu des années 1950, avec les débuts de l'informatique. Mais l'idée de "machines pensantes" est une quête mythologique et philosophique depuis l'aube de l'humanité. Cet héritage a brouillé notre compréhension de ce que l'on peut attendre d'une machine "intelligente".
S'en tenir au comportement factuel d'une IA, à sa finalité et à ses applications pratiques permet de dépasser les mythes. L'intelligence artificielle décrit en fait une intelligence artificielle qui imite les fonctions "cognitives" que les humains associent à l'esprit humain, telles que l'"apprentissage" et la "résolution de problèmes".
Cela signifie que les applications commerciales de l'IA ne peuvent découler que d'une identification et d'une compréhension claires du problème à résoudre. Car une machine n'a pas (encore) d'"esprit propre" pour décider d'appliquer ses compétences à la résolution de problèmes !
Exemples de résolution de problèmes avec l'IA et les agents virtuels
L'intelligence artificielle permet déjà de résoudre des problèmes dans de nombreux domaines, notamment les transports (véhicules autonomes), la santé (détection des cellules cancéreuses à un stade précoce), l'agriculture (surveillance des cultures et des sols), la finance (analyse des marchés et exploration des données) et l'armée (collecte de données de renseignement).
L'une des applications les plus intéressantes des technologies d'apprentissage automatique de l'IA sont les Agents virtuels intelligents. Ces robots conversationnels sont capables d'interagir avec les humains grâce à la compréhension du langage. Les agents virtuels peuvent engager une conversation avec les utilisateurs par l'intermédiaire d'un chat en direct, d'un courrier électronique, mais aussi par la voix. Ils comprennent le langage humain et les intentions qui se cachent derrière les demandes.
L'enjeu est ici de générer des gains de productivité et d'améliorer la satisfaction des utilisateurs.
Le cas le plus courant est de permettre aux utilisateurs de résoudre un problème de réinitialisation de mot de passe ou de verrouillage de compte, en discutant avec un agent virtuel intelligent ou en l'appelant. Étant donné que 40 % des interventions des centres d'appel concernent des problèmes de mots de passe (selon Gartner), laisser un agent virtuel s'en occuper peut faire gagner du temps et du budget au service d'assistance IT.
Les compétences conversationnelles avancées et la compréhension de l'intention permettent également à un agent virtuel de traiter des demandes IT plus complexes et de résoudre des problèmes. Cette capacité à effectuer une analyse sémantique, à comprendre l'incident d'un utilisateur et à le résoudre est au cœur de la mission du service d'assistance IT.
Mais d'abord, l'agent virtuel doit être capable de comprendre si le problème de l'utilisateur relève ou non de son domaine d'expertise. Pour ce faire, il fait correspondre la demande avec ses bases de connaissances, ses FAQ et le contenu de l'entreprise auquel il a été autorisé à accéder.
Ensuite, l'agent virtuel peut guider l'utilisateur à travers une procédure complexe dans le cadre d'une conversation (par exemple, des demandes pour "se connecter à un VPN" ou "libérer de l'espace sur le disque dur"). L'agent peut également proposer des choix et clarifier les déclarations vagues ou imprécises de l'utilisateur.
Si la demande sort du cadre de son programme informatique, l'agent virtuel peut tout de même proposer des solutions pour aider les utilisateurs à progresser dans leurs demandes. Par exemple, il peut proposer d'envoyer un courriel à un contact RH pour une question relative aux ressources humaines.
Nous voyons ici que la compréhension des langues et des intentions permet à l'agent virtuel de faire plus que d'aller chercher une réponse dans une base de données.
L'idée est d'engager une conversation qui coule de source, d'adapter les réponses aux demandes et au contexte de l'utilisateur. Il est également important de rassurer les utilisateurs à chaque étape du processus, afin de garantir leur satisfaction.
Concentrons-nous à présent sur la technologie qui soutient ces compétences conversationnelles : l'apprentissage machine et l'apprentissage profond.
La différence entre l'apprentissage automatique et l'apprentissage profond
L'apprentissage automatique (Machine Learning) est un sous-ensemble de l'intelligence artificielle. L'apprentissage automatique est l'étude des algorithmes informatiques qui s'améliorent automatiquement grâce à l'expérience et à l'entraînement sur des données, grâce à des modèles d'apprentissage et à des techniques d'apprentissage. .
Les agents virtuels intelligents utilisent un sous-ensemble de l'apprentissage automatique appelé apprentissage profond. L'apprentissage profond est basé sur les réseaux neuronaux, dont le modèle s'inspire librement du cerveau humain qui fonctionne avec une superposition de neurones, et qui sont utilisés pour détecter des modèles.
Cette approche des réseaux neuronaux pour l'apprentissage automatique profond est la base du traitement du langage naturel (NLP), de la compréhension (NLU) et de la génération de langage naturel (NLG).
Comment les agents virtuels comprennent-ils le le langage naturel
Quels sont les schémas que les réseaux neuronaux peuvent identifier au cours d'une conversation écrite ou vocale avec un être humain ? Les modèles sont des "similitudes sémantiques", des combinaisons reconnaissables de mots et de phrases que l'agent virtuel utilisera pour comprendre la requête d'un utilisateur et poursuivre une communication basée sur l'intention.
L'agent virtuel peut avoir besoin de poser des questions supplémentaires pour comprendre réellement ce que l'utilisateur veut dire ou ce dont il a besoin, et de faire correspondre cette demande avec des réponses provenant de ses bases de connaissances.
Couplé à la RPA (Robotic Process Automation), l'agent virtuel peut même orchestrer des actions multiples comme l'envoi de courriels ou la génération de mots de passe, de codes Wi-Fi...
Car l'idée n'est pas de planifier et de classer toutes les requêtes possibles pouvant provenir d'un être humain. L'objectif est de créer un moteur NLU-NLG qui améliorera sa compréhension des conversations humaines au fil du temps grâce à des algorithmes d'apprentissage. Il apprendra à partir d'une grande variété de sources de données telles que des bases de connaissances existantes ou des conversations réelles avec des utilisateurs.
L'apprentissage profond comme fondement de la croissance et du changement
La force du modèle d'apprentissage profond vient de sa capacité à apprendre à partir de son ensemble de données et à accepter une grande variété de données.
Il permet à un agent virtuel de comprendre les questions et d'y répondre, mais aussi de gérer les changements de contexte et les digressions pour une meilleure reconnaissance vocale lors d'une conversation avec un humain. Cela signifie que même si un utilisateur saute certaines questions, l'agent virtuel ne sera pas bloqué dans un scénario mais s'adaptera en temps réel à la conversation.
C'est pourquoi les agents virtuels sont considérés comme une "main-d'œuvre numérique" qui travaille en partenariat avec les humains. Dans les environnements informatiques et de travail numérique d'aujourd'hui (comme Microsoft 365) où de nouveaux outils et logiciels sont régulièrement déployés, les utilisateurs finaux doivent s'adapter rapidement à la transformation numérique. La courbe d'apprentissage est très rapide pour un agent virtuel déjà entraîné sur des bases de connaissances (telles que Microsoft ou ServiceNow) et aux conversations réelles avec les utilisateurs finaux.
Chez Konverso, nous appliquons l'intelligence artificielle à un domaine que nous connaissons bien : le Service Desk IT et les environnements de travail numériques.